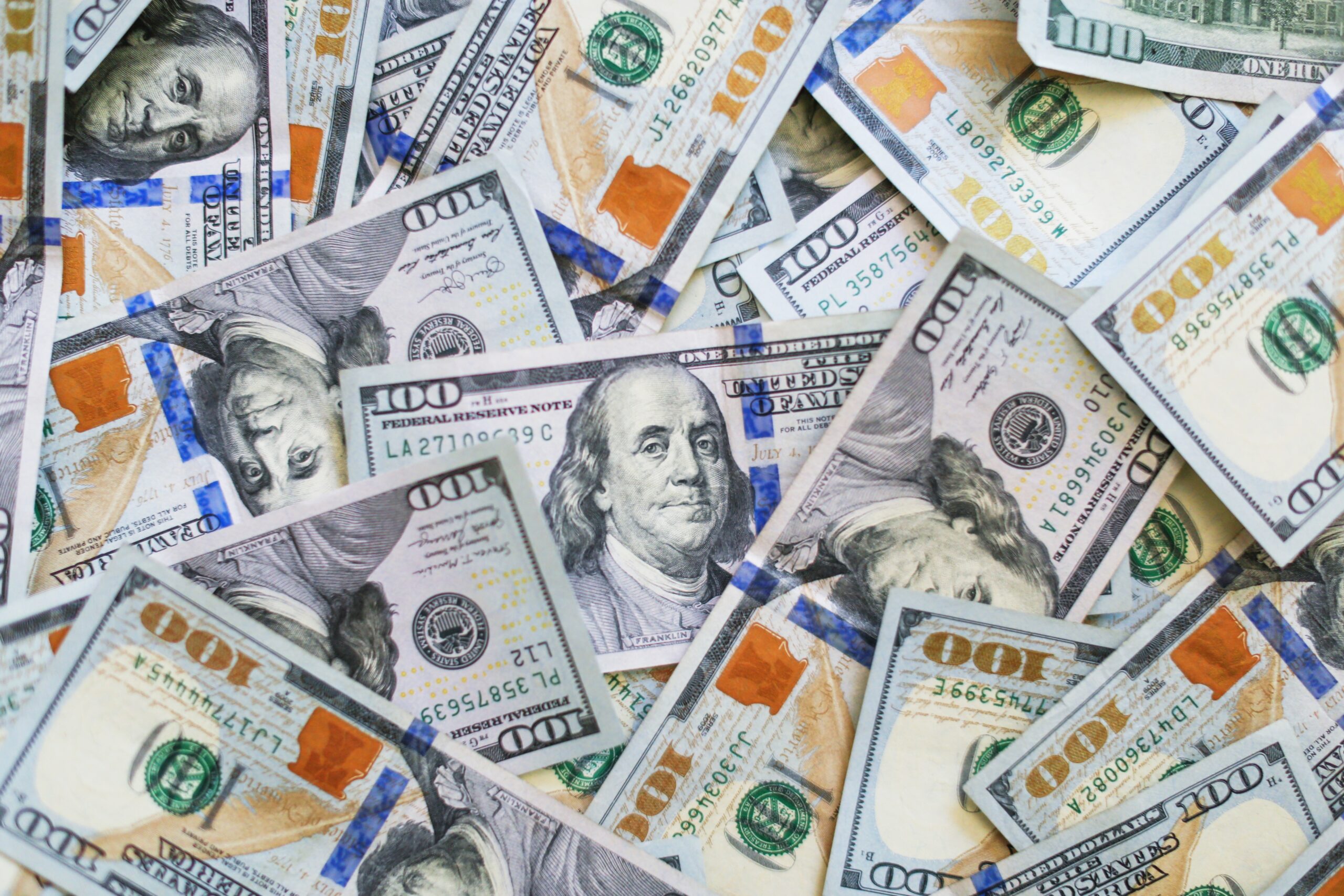
In the fast-paced world of customer service, finding efficient and effective ways to meet the demands of your clients is crucial. That’s where GPT-driven customer service comes in. By harnessing the power of advanced language models, businesses can automate their customer interactions to provide seamless support and improve productivity. But what sets GPT-driven customer service apart is its potential for generating revenue. In this article, we will explore how integrating GPT technology can not only streamline your customer service operations but also help you make money along the way. So buckle up, because it’s time to automate to elevate!
Heading 1: Understanding GPT-Driven Customer Service
Subheading 1: The concept of GPT-Driven Customer Service
GPT-Driven Customer Service refers to the use of Generative Pre-training Transformers (GPT) models to automate and enhance customer service interactions. GPT models are designed based on deep learning techniques and can generate human-like responses based on given prompts. By applying this technology to the customer service field, businesses can provide faster and more efficient support to their customers.
Subheading 2: Benefits of using GPT-Driven Customer Service
There are several benefits to implementing GPT-Driven Customer Service. Firstly, it enables businesses to provide round-the-clock support to their customers without the need for human intervention. This ensures that customers can receive assistance whenever they need it, improving their overall satisfaction and loyalty.
Secondly, GPT-Driven Customer Service can handle a large volume of customer queries simultaneously, reducing wait times and improving response times. This capability allows businesses to handle spikes in customer inquiries efficiently, especially during peak periods.
Lastly, GPT-Driven Customer Service can reduce the operational costs associated with customer support. By automating repetitive and simple inquiries, businesses can free up their customer service agents to focus on more complex and critical tasks. This optimization of resources can result in significant cost savings for the organization.
Subheading 3: How GPT-Driven Customer Service works
GPT-Driven Customer Service works by training a GPT model on a vast amount of customer service data. This training data includes examples of customer queries and corresponding responses from human customer service agents. The GPT model learns from this data and gains the ability to generate relevant and accurate responses to new customer queries.
When a customer submits a query, the GPT model processes the input and generates a response based on its training and understanding of the given prompt. This response is then delivered to the customer through the chosen customer service channel, such as chatbots, virtual assistants, or automated email systems. The entire process is designed to seamlessly mimic human-like interactions and provide satisfactory solutions to customer inquiries.
Heading 2: Choosing the Right GPT Platform
Subheading 1: Researching available GPT platforms
When considering GPT platforms for customer service automation, it is important to conduct thorough research on the available options. There are several GPT platform providers in the market, each offering different features and capabilities. By researching and evaluating these platforms, businesses can make informed decisions about which platform best suits their specific needs.
Subheading 2: Comparing features and capabilities
When comparing GPT platforms, it is essential to consider the features and capabilities they offer. Some platforms may have built-in natural language understanding (NLU) capabilities, enabling more accurate responses to customer queries. Others may offer advanced customization options, allowing businesses to tailor the GPT model to their specific industry or use case. By comparing the available features, businesses can select a platform that aligns with their objectives and requirements.
Subheading 3: Determining cost and scalability
Cost and scalability are also crucial factors to consider when choosing a GPT platform for customer service automation. Businesses should assess the pricing models of different platforms and determine if they align with their budgetary constraints. Additionally, evaluating the scalability of a platform is essential to ensure that it can handle a growing volume of customer service queries as the business expands. It is important to choose a platform that can accommodate future growth without compromising performance or incurring excessive costs.
Heading 3: Training the GPT Language Model
Subheading 1: Preparing training data for the GPT model
To train the GPT model effectively, businesses must prepare high-quality training data. This data should consist of customer service interactions and their corresponding responses. It is crucial to include a diverse range of scenarios and examples to ensure the model’s ability to handle various customer queries.
Subheading 2: Fine-tuning the model for customer service
After preparing the training data, businesses can fine-tune the GPT model specifically for customer service. This process involves training the model on the prepared data to enhance its understanding of customer inquiries and improve the accuracy of its responses. Fine-tuning allows the model to adapt to the specific nuances and requirements of the customer service domain, leading to more effective and contextually appropriate interactions.
Subheading 3: Monitoring and iterating the training process
Training a GPT model for customer service is an ongoing process that requires continuous monitoring and iteration. Businesses should evaluate the model’s performance regularly and make necessary adjustments to improve its accuracy and response quality. This iterative approach allows the GPT model to continuously learn and evolve, ensuring its suitability for the changing needs of customer service over time.
Heading 4: Integrating GPT-Driven Customer Service
Subheading 1: Identifying customer touchpoints for automation
Implementing GPT-Driven Customer Service requires identifying the customer touchpoints that can benefit from automation. Businesses need to analyze the customer journey and identify interactions that can be efficiently and effectively handled by the GPT model. This analysis may involve identifying repetitive or frequently asked questions that can be automated, freeing up human agents to focus on more complex inquiries.
Subheading 2: Developing APIs and integrations
Once the customer touchpoints for automation are identified, businesses should develop APIs and integrations to seamlessly connect the GPT model with their existing customer service systems. These integrations allow for the smooth transfer of customer data, inquiries, and responses between the GPT model and other customer service channels. Well-designed APIs and integrations are crucial to ensure a cohesive and efficient customer service experience.
Subheading 3: Testing and refining the integration
Before deploying GPT-Driven Customer Service, thorough testing and refinement of the integration are necessary. This process involves conducting extensive testing to identify any potential issues or bugs in the system. It is essential to ensure that the integration functions as intended and delivers accurate and reliable responses to customer inquiries. Testing and refinement iterations are essential for achieving a seamless integration that enhances the overall customer service experience.
Heading 5: Ensuring Data Security and Privacy
Subheading 1: Understanding privacy concerns with GPT-driven systems
GPT-Driven Customer Service systems deal with sensitive customer data, making data security and privacy of paramount importance. As customer inquiries are processed and stored, businesses must ensure that proper privacy protocols are in place to protect this information. It is crucial to address potential privacy concerns and design systems that prioritize customer data protection.
Subheading 2: Implementing strong data security measures
To ensure data security, businesses should implement robust measures across their GPT-Driven Customer Service systems. Encryption and secure storage methods should be employed to prevent unauthorized access to customer data. Additionally, access controls should be established to restrict data access only to necessary personnel. Regular security audits and compliance checks are also crucial to maintain a secure environment for customer information.
Subheading 3: Complying with data protection regulations
Compliance with data protection regulations is essential for businesses utilizing GPT-Driven Customer Service. Depending on the jurisdiction, different regulations such as the General Data Protection Regulation (GDPR) may apply. Businesses must ensure that their data handling practices align with these regulations and that customers’ privacy rights are respected. Compliance demonstrates a commitment to data protection and enhances customer trust in the organization.
Heading 6: Optimizing GPT-Driven Customer Service
Subheading 1: Analyzing customer interactions and feedback
Optimizing GPT-Driven Customer Service requires a thorough analysis of customer interactions and feedback. Businesses should monitor and analyze the interactions between customers and the GPT model to identify areas for improvement. By understanding customer needs and pain points, businesses can tailor the GPT model to deliver more accurate and satisfactory responses.
Subheading 2: Identifying areas for improvement
Based on the analysis of customer interactions and feedback, businesses can identify areas within the GPT-Driven Customer Service system that require improvement. This may involve refining the training data, adjusting the fine-tuning process, or updating the model architecture. Identifying and addressing these areas for improvement allows businesses to continuously enhance the performance of the GPT system and deliver better customer experiences.
Subheading 3: Regular model updates and retraining
To ensure the optimal performance of GPT-Driven Customer Service, regular model updates and retraining are necessary. As the customer service landscape evolves, the GPT model needs to adapt to changing customer needs and expectations. Continuous updates and retraining of the model based on new data and insights enable businesses to stay ahead of customer service trends and provide the best possible support to their customers.
Heading 7: Managing Customer Expectations
Subheading 1: Setting realistic expectations for AI-powered customer service
Managing customer expectations is vital when implementing AI-powered customer service. While GPT-Driven Customer Service can provide quick and accurate responses, it is essential to set realistic expectations about its limitations. Communicating that the customer is interacting with an AI system and explaining its capabilities and potential areas of uncertainty can manage customer expectations and avoid frustration.
Subheading 2: Training customer service agents to collaborate with GPT
Collaboration between customer service agents and the GPT model is key to successful implementation. Businesses should invest in training their customer service agents to collaborate effectively with the GPT system. This training can include understanding its capabilities, managing handoff procedures, and providing necessary oversight and assistance to ensure a smooth customer service experience.
Subheading 3: Addressing limitations and fallback options
Despite the advancements in GPT technology, limitations and fallback options should be considered. There may be instances where the GPT model cannot provide satisfactory responses or encounters ambiguous or complex queries. In such cases, businesses should have fallback options, such as the ability to transfer the inquiry to a human agent, to ensure customer satisfaction. Addressing these limitations proactively and having contingency plans in place can maintain customer trust and prevent dissatisfaction.
Heading 8: Measuring Success and ROI
Subheading 1: Defining metrics for success
To measure the success of GPT-Driven Customer Service, businesses should define relevant metrics. These metrics may include customer satisfaction scores, response times, resolution rates, and cost savings achieved through automation. Defining clear metrics allows businesses to assess the effectiveness of their GPT-Driven Customer Service implementation and track its impact on customer service operations.
Subheading 2: Tracking customer satisfaction and response times
Customer satisfaction and response times are critical metrics for measuring the success of GPT-Driven Customer Service. Regularly tracking and analyzing customer satisfaction levels provides insights into the overall quality and effectiveness of the system. Monitoring response times also allows businesses to identify potential bottlenecks and areas for improvement to ensure timely and efficient customer support.
Subheading 3: Evaluating cost savings and revenue generation
GPT-Driven Customer Service can bring significant cost savings to businesses by automating repetitive and low-complexity tasks. Evaluating the cost savings achieved through GPT automation is crucial to understand the return on investment (ROI) of implementing this technology. Additionally, businesses should also assess any indirect revenue generation resulting from improved customer satisfaction and loyalty. This comprehensive evaluation helps quantify the economic benefits of GPT-Driven Customer Service and justifies the investment made.
Heading 9: Overcoming Challenges in GPT-Driven Customer Service
Subheading 1: Handling ambiguous customer queries
One of the challenges in GPT-Driven Customer Service is handling ambiguous customer queries. GPT models sometimes struggle with understanding the context or nuances of certain inquiries, resulting in less accurate responses. To overcome this challenge, businesses can implement techniques such as providing clarifying prompts or utilizing fallback options to involve human agents when necessary. Continuous monitoring and improvement of the GPT model’s training can also enhance its ability to handle ambiguous queries more effectively.
Subheading 2: Dealing with language nuances and understanding context
Language nuances and contextual understanding can pose challenges for GPT-Driven Customer Service. GPT models may struggle with understanding idiomatic expressions, slang, or industry-specific terminology. To address this challenge, businesses can invest in training the GPT model on domain-specific data and utilizing Natural Language Processing (NLP) techniques to improve contextual understanding. Combining the strengths of AI with human oversight and intervention can help overcome these language and contextual challenges.
Subheading 3: Avoiding bias and ethical considerations
Bias and ethical considerations are important challenges to address in GPT-Driven Customer Service. GPT models learn from the data they are trained on, and if this data contains biases or unethical content, it can be reflected in the model’s responses. To mitigate this, businesses should ensure that the training data is diverse and representative to avoid reinforcing any existing biases. Regular audits and reviews of the GPT model’s responses can identify and rectify any potential biases, ensuring fair and ethical customer service interactions.
Heading 10: Future Trends and Considerations
Subheading 1: Advancements in GPT technology
The field of GPT-Driven Customer Service is continuously evolving, with advancements in GPT technology expected in the future. These advancements may include improved contextual understanding, better handling of language nuances, and enhanced natural language generation capabilities. Monitoring and adapting to these technological advancements can help businesses stay ahead and provide cutting-edge customer service experiences.
Subheading 2: AI-human collaboration in customer service
The future of customer service is likely to involve increased collaboration between AI systems and human agents. While GPT-Driven Customer Service can automate many inquiries, there will always be cases that require human intervention. Developing systems that seamlessly integrate AI and human support can provide the best of both worlds, combining the efficiency and scalability of AI with the empathetic and problem-solving capabilities of human agents.
Subheading 3: Ethical implications and automated decision-making
As GPT-Driven Customer Service becomes more prevalent, ethical implications of automated decision-making need to be carefully considered. There may be scenarios where GPT models play a role in making critical decisions that impact customers. Businesses must ensure transparency, fairness, and accountability in their automated decision-making processes. Regular ethical evaluations and adhering to ethical guidelines in AI development can help navigate these considerations and build trust with customers.
In conclusion, GPT-Driven Customer Service offers businesses the opportunity to automate and enhance their customer service interactions. By selecting the right GPT platform, training the GPT language model effectively, integrating automation into customer touchpoints, and ensuring data security and privacy, businesses can elevate their customer service capabilities. Continuous optimization, managing customer expectations, measuring success, and overcoming challenges are key elements to consider. By staying informed about future trends and ethical implications, businesses can embrace the benefits of GPT-Driven Customer Service and deliver exceptional customer experiences.